Math and data say early-stage VCs should index invest, and late-stage investors should stock pick.
Yet they do the opposite.
The reason is counterintuitive: VCs are picky, not because they have so many options but because they have so few.
More deals, better performance
Theoretical modeling and empirical data both suggest that early-stage VCs do better when they spread the wealth wider.
Let's start with the theory.
First, we need to define the power law distribution:
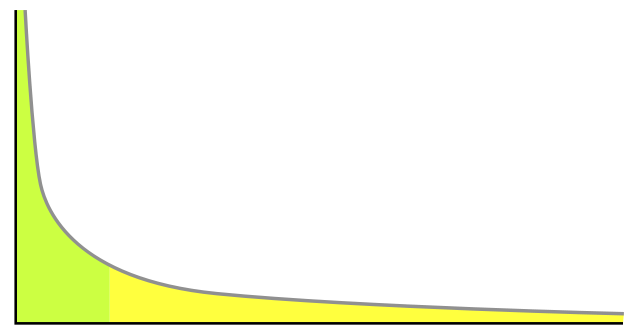
A power law distribution is one where large, consequential events (the Googles, Amazons, and Facebooks of the venture world) are rare but much more common than you might expect if the world were normally distributed.
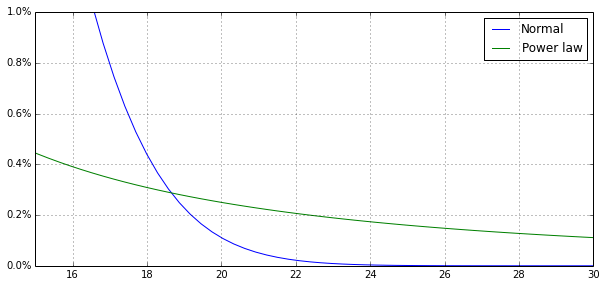
These events happen with some frequency, and that frequency can be characterized by a "shape" or "tail parameter" that governs the "fatness" of the tail, typically represented by the symbol \(\alpha\).
The smaller \(\alpha\), the "fatter" the tail of the power law distribution. The fatter the tail, the higher the frequency and size of outlier startups.
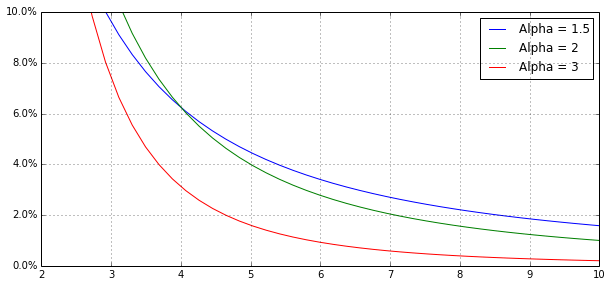
If \(\alpha\) is sufficiently small, strange things begin to happen. With a small enough \(\alpha\), making investments nearly at random will increase a portfolio's expected return.
The exact mathematical reasons for this are somewhat esoteric, but intuitively, if there are enough potential Googles, Amazons, and Facebooks out there, they will more than cover the losses from the duds thanks to the shape of the power law distribution. When fat tails dominate returns it's not worth having an extremely high bar if it might cause you to miss out on one of these future juggernauts.
VCs often talk about power laws, but few have sufficient data to rigorously demonstrate them. However, AngelList does, and their head data scientist, Abe Othman, has done the work to analyze returns from the thousands of deals syndicated by AngelList, finding that:
... the regret an investor could have for missing a winning seed-stage investment is theoretically infinite, a phenomenon that does not appear to hold for later-stage investments. The implication is that investors increase their expected return by indexing as broadly as possible at the seed stage (i.e., by putting money into every credible deal), because any selective policy for seed-stage investing—absent perfect foresight—will eventually be outperformed by an indexing approach.
By creating power law-based mathematical model, fitting it to the AngelList returns data, and simulating 50,000 portfolios with 10 early-stage companies each, he created the following synthetic distribution of venture capital returns:
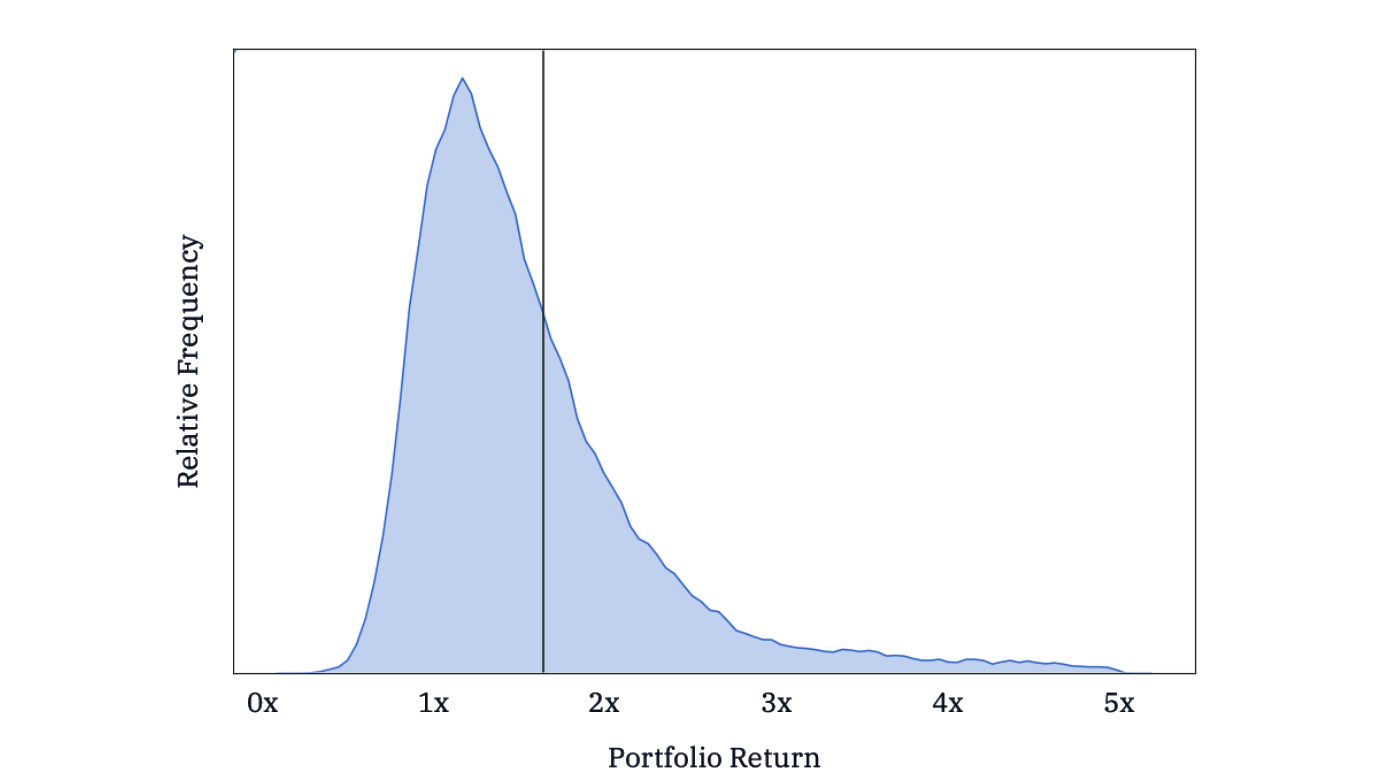
The vertical black line represents the market return: the return you'd earn if you invested an equal amount in every AngelList deal in the sample (of which there were 1,808). Notice the long tail trailing off to the right side of the chart. In a world where most barely return their fund, some shower their LPs with 5x returns. Notice how most 10-company portfolios underperform the simple (1,808-company) strategy.
Technically, this is still a theoretical result. Yes, the model was fit to real data, but the outputs are still simulated. Now let's look at real data.
Here again we turn to AngelList, who analyze the relationship between portfolio size and performance among 10,000+ investors on the platform. They grouped the investors by number of companies in their portfolio and plotted the median return for each group. The chart confirms the theoretical results - larger portfolios generate greater returns:
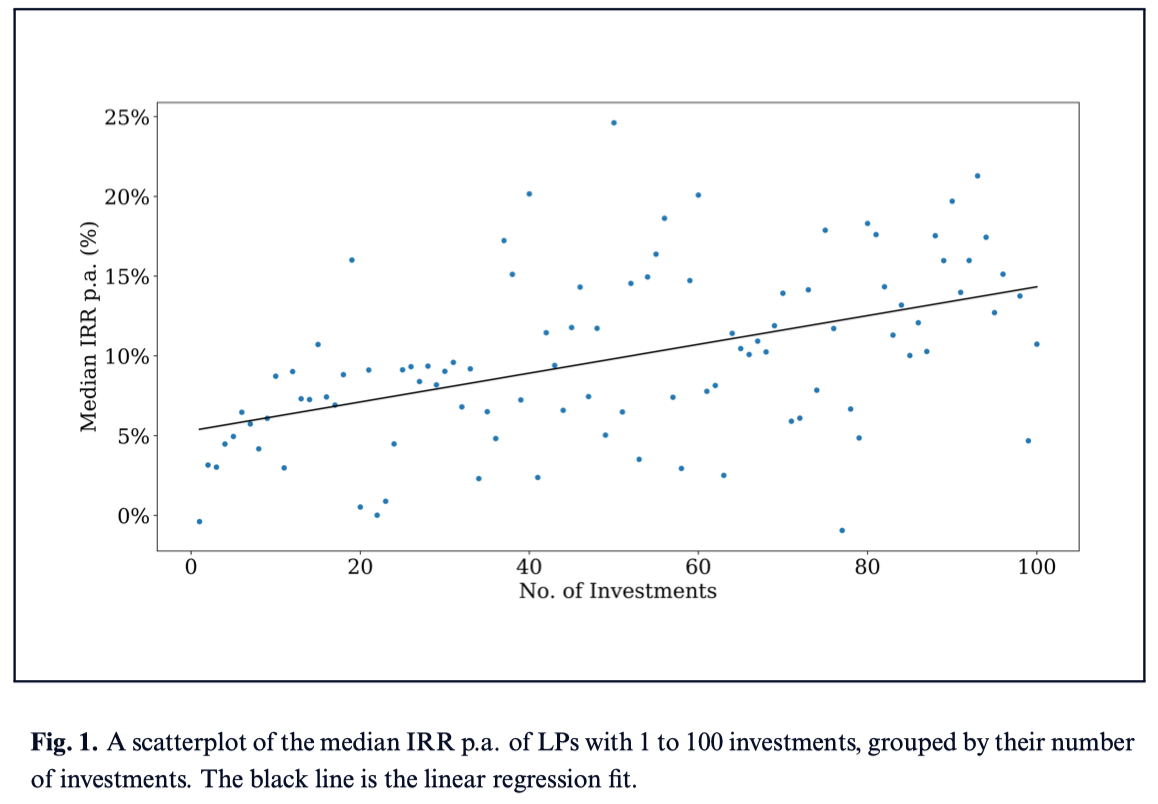
The coefficient of the regression term is 9.0 basis points... implying that the typical annual return of a portfolio of 100 investments is almost 9% higher than the typical annual return of a portfolio with a single investment.
Note these are median returns, so they don't reflect a handful of 100-count portfolios simply getting lucky and pulling up the average. Financial upside is easier to come by when you're exposed to a larger patch of the startup landscape.
Theory and data agree - most early-stage venture investors would do better by indexing, investing a small amount in every reasonable startup they can find:
Simulations on 10-year investing windows for seed-stage deals suggest fewer than 10% of investors will beat the index, even if those investors have skill in picking deals. Like Vanguard has taught us in the public markets, individual investors could benefit from viewing the index as the default and then overlaying individual deals that they like.
1/N
Behavioral finance theorists have a name for this strategy: the 1/N heuristic.
It goes like this: take every investable asset of a group of N assets and invest 1/Nth of your capital in each one, with no regard to the fundamentals, mean return, or volatility of returns.
This might sound like a dumb, simplistic strategy. While it's certainly simple, it's definitely not dumb.
Ironically, 1990 Nobel Laureate and famed inventor of mean-variance portfolio optimization, Harry Markowitz, reportedly used this exact heuristic for investing his own money, eschewing his own complicated theory in favor of a simpler approach:
I should have computed the historical covariance of the asset classes and drawn an efficient frontier…I split my contributions 50/50 between bonds and equities. - Harry Markowitz
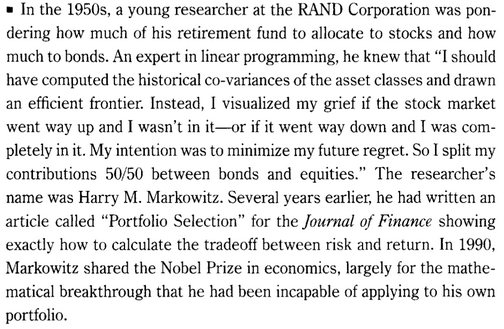
In fact, one study found that the 1/N strategy dominates numerous others:
Of the 14 models we evaluate across seven empirical datasets, none is consistently better than the 1/N rule in terms of Sharpe ratio, certainty-equivalent return, or turnover, which indicates that, out of sample, the gain from optimal diversification is more than offset by estimation error... That is, the effect of estimation error is so large that it erodes completely the gains from optimal diversification.
Which is a fancy way of saying "don't overcomplicate it."
A slightly more sophisticated reading of the results might be, "unless you are very sure about the structure of the world, don't overcomplicate your investing strategy."
The study found a negative return to overthinking and overfitting yourself to the past data, to what you think you know about these assets. In venture, similar overintellectualization has been the root cause of numerous "misses" over the years - career, fund, and firm-defining deals that put certain VCs on the map and left others in desperate obscurity.
In some subtle way, trying to pick the exact right startup based on a complex model of the world is like trying to buy a certain lottery ticket with a certain serial number because you think it's a "lucky ticket." One seems much more superstitious, but are they closer than they appear?
How the other half lives
Late stage investors on the other hand, can afford to stock pick:
... our results also suggest that the opportunity cost for missing a winning investment in these later rounds is bounded... Consequently, it is entirely appropriate that later-stage investors should reject the “spray and pray” idea and be thoughtful and discerning when they participate...
Translation: late-stage deals have lower opportunity cost than early-stage investments, as their returns are capped much lower. To channel Jeff Bezos' regret minimization framework: there isn't much regret to minimize when it comes to late-stage deals. So feel free to be picky.
Another take from the 1/N study:
... we conclude that portfolio strategies from the optimizing models are expected to outperform the 1/N benchmark if: (i) the estimation window is long; (ii) the ex ante (true) Sharpe ratio of the mean-variance efficient portfolio is substantially higher than that of the 1/N portfolio; and (iii) the number of assets is small.
In other words, it makes sense to be picky and particular when you have a large amount of data collected over a long period of time and when the number of choices is small. Both are more true of later-stage investments relative to early-stage. By definition, late-stage companies have a longer track record by which to evaluate them, and there are fewer of them. Thus, it makes sense to be a discerning capital allocator at the late-stage.
And yet we've seen the exact opposite in recent years.
Though few would publicly characterize themselves as such, I know of at least a few firms who have explicit goals of creating, effectively, "indices of late-stage venture" via their large portfolios. Late-stage funds are raising and deploying capital at unprecedented rates. Many appear principally focused on putting "dollars to work," rather than earning the maximum return on their capital. They've taken to heart the approach discussed earlier, investing in every company that meets some minimum threshold.
This has led to serious perversion at the late-stage, as there are not nearly enough investable assets to support the capital inflows at reasonable valuations. Asset values grow not simply due to improvement in fundamental startup value but also because late-stage funds need somewhere to park their money.
In this model, late-stage startups become "capital vehicles," absorbing meaningful capital and earning a "capital storage" premium for their efforts.
Party rounds, long known to greatly inflate early-stage valuations, have reached the late-stage.
But all parties must come to end eventually...
Caveat emptor indexor
So that's the theory, but there are some real practical hurdles to creating an early-stage index:
- Determining the right minimum threshold
- No one sees every deal
- Deals must be won
- Fund and check size restrictions
How high should the bar be?
AngelList recommends investing in every credible early-stage deal, where the word "credible" does a lot of work. They add:
We worry that an investor promising to blindly fund every whisper of a new company would fundamentally alter the investment universe they are exposed to by introducing a huge number of new money losing investments that otherwise would not have been created but for the investor’s universal funding policy. Consequently, our results suggest that at the seed stage investors should put money into every investment that clears some minimum threshold.
But how to set that threshold? It's not obvious.
There must be some bar, even if very low, otherwise you'll do a series of bad deals and get fleeced.
Peter Thiel puts it this way:
There just aren’t that many businesses that you can have the requisite high degree of conviction about. A better model is to invest in maybe 7 or 8 promising companies from which you think you can get a 10x return.
Part of the reason indexing even works in say, public markets, is that there is some minimum threshold a company has to reach to even go public. Additionally, the AngelList data from which this theory comes only includes deals that got done, by definition. We don't know if the indexing strategy carries over to deals that wouldn't have otherwise got done or to companies with lower quality than the typical AngelList deal.
Since the AngelList data comes from deals that successfully completed, you could proxy for this by setting the bar such that you would do any deal that some other reasonable person would do.
You must see the deal to do the deal
You can't hit a target you can't see, and you can't do a deal you can't see either.
The AngelList recommendation assumes the investor could invest in any deal they want. If you don't have access to all the deals, this logic breaks down.
Unless you are a top tier fund or individual investor, the specific subset of deals you see is almost certainly poor relative to the full distribution. You are likely missing some serious outliers.
This is why so many VCs spend so much time burnishing their public and private reputations. VC Twitter says hi.
You must also win
Let's say you set the right threshold and see all the deals that meet it. You still then must win the deal, which is far from guaranteed.
Further, the greater extent to which a deal exceeds any given threshold, the more competitive the deal will be. Assuming there's signal there and not simply noise, your portfolio will probabilistically skew lower quality simply due to losing out on those winners, even though you recognized them as such.
This completely screws up the indexing strategy, which implicitly relies on free and open access to all deals across the quality spectrum.
A fund cannot be sliced into a thousand pieces
Even if the above caveats don't apply, a VC still runs up against fundamental limits of the venture universe.
Like the Planck length represents the smallest distance at which conventional physics applies, micro-checks become infeasible below a certain size, both for the startups themselves and the would-be index investor.
The complement of this is that, assuming some minimum check size, you might not be able to raise a fund large enough to write all those checks.
Masters of the universe?
VCs are picky, not because they have so many options but because they have so few.
The 1/N study tells us that stock picking makes sense when there are few options to choose from.
The AngelList data tells us that, in a hypothetical world of perfect access to all investments, you'd do better to spread your bets widely.
In other words, the picky behavior of early-stage venture capitalists in light of the data and theory around power laws can only be justified by their lack of options. They only have a few shots on goal and must use them wisely.
People often assume this pickiness comes from the fact that most startups fail, and VCs obviously want to avoid the numerous duds. But this isn't really true. Even in a world where most startups fail it can make sense to invest in many more than the typical investor does currently, as shown above.
Far from masters of the universe, selectively picking among desperate founders looking for funding, VCs are themselves starved of options, making them extremely cautious with the few they have.
In a future article, I'll extend this idea and demonstrate why it shows venture capitalists aren't funding nearly enough startups.